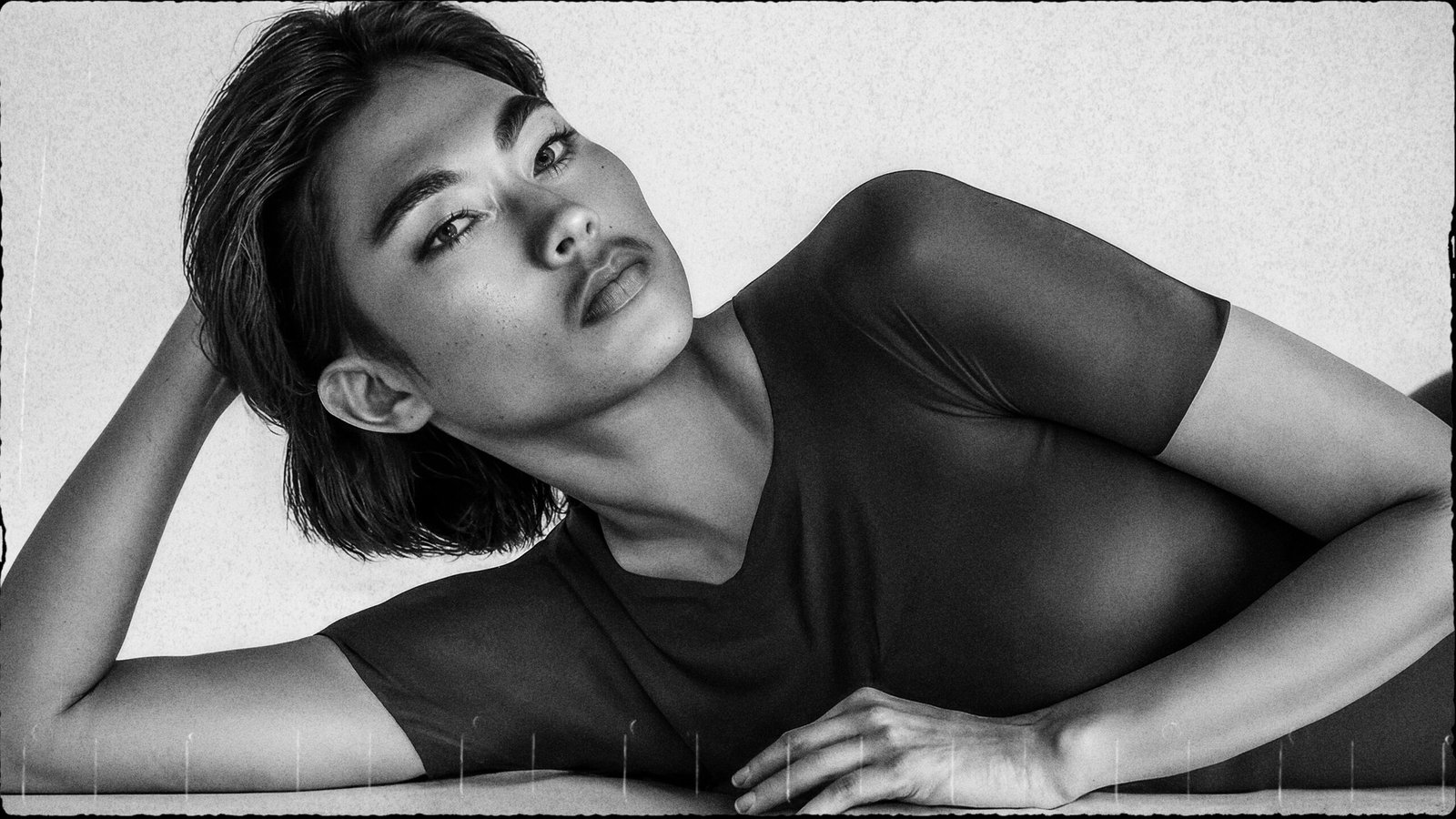
What is AI: Understanding the Basics of Artificial Intelligence
Introduction to Artificial Intelligence
Artificial Intelligence (AI) is a branch of computer science focused on creating systems capable of performing tasks that typically require human intelligence. These tasks include learning, reasoning, problem-solving, perception, and language understanding. The term “Artificial Intelligence” was first coined by John McCarthy in 1956, marking the beginning of a new era in technological advancement.
The history of AI is marked by several key milestones. The inception of AI research can be traced back to the mid-20th century. Early pioneers like Alan Turing laid the foundations, proposing the Turing Test as a measure of machine intelligence. Through the decades, AI has evolved significantly, with notable advancements such as IBM’s Deep Blue defeating chess champion Garry Kasparov in 1997 and, more recently, Google’s AlphaGo mastering the complex game of Go.
Fundamental concepts in AI include machine learning, neural networks, and natural language processing. Machine learning, a subset of AI, involves training algorithms on large datasets to enable them to make predictions or decisions. Neural networks, inspired by the human brain, consist of interconnected nodes that process information in layers. Natural language processing allows machines to understand and generate human language, facilitating advancements in communication technologies.
AI can be categorized into three types: Narrow AI, General AI, and Superintelligent AI. Narrow AI, also known as weak AI, is designed for specific tasks such as speech recognition or facial recognition. General AI, or strong AI, aims to perform any intellectual task that a human can do, though it remains largely theoretical at this stage. Superintelligent AI refers to a level of intelligence surpassing that of the brightest human minds, a concept that is still in the realm of speculation and science fiction.
Understanding these foundational elements of AI is essential for grasping its current applications and future potential. As AI continues to integrate into various aspects of our lives, its importance and impact on society will only grow.
Types and Applications of AI
Artificial Intelligence (AI) is broadly categorized into three types: Narrow AI, General AI, and Superintelligent AI. Narrow AI, also known as Weak AI, is designed to perform specific tasks. These systems do not possess general intelligence but excel in their designated functions. For instance, virtual assistants like Siri and Alexa, recommendation algorithms used by Netflix and Spotify, and image recognition software in social media are exemplary applications of Narrow AI. These systems are tailored to handle specific problems efficiently, making them highly effective in their respective domains.
General AI, or Strong AI, represents a more advanced form of artificial intelligence, with the capability to perform any intellectual task that a human can do. While Narrow AI operates within predefined parameters, General AI aims to understand, learn, and apply knowledge across a wide range of tasks. Although true General AI is still in the theoretical and developmental stages, it remains a significant focus of research, with the potential to revolutionize various aspects of human life and industry.
Superintelligent AI is a theoretical concept referring to an AI that surpasses human intelligence across all fields, including creativity, problem-solving, and emotional intelligence. While this form of AI remains speculative, its potential implications are the subject of extensive debate among researchers and ethicists, particularly concerning its impact on society and ethical considerations.
The applications of AI are vast and span multiple industries. In healthcare, AI is utilized for predictive analytics, personalized medicine, and robotic-assisted surgeries, enhancing diagnosis accuracy and treatment efficacy. In the finance sector, AI algorithms are employed for fraud detection, risk assessment, and algorithmic trading, improving financial security and market efficiency. The transportation industry benefits from AI through autonomous vehicles and smart traffic management systems, which aim to reduce accidents and optimize traffic flow. Entertainment also leverages AI for content creation, gaming, and personalized recommendations, providing enhanced user experiences.
Overall, AI’s ability to solve complex problems and improve efficiency makes it an invaluable asset across various sectors, continually pushing the boundaries of what technology can achieve.
How AI Works: Key Technologies and Techniques
Artificial Intelligence (AI) operates through a synergy of several core technologies and techniques that enable it to perform tasks requiring human-like intelligence. At the heart of AI are machine learning, deep learning, neural networks, and natural language processing. Each of these components plays a crucial role in creating intelligent systems capable of learning, reasoning, and adapting.
Machine Learning (ML): Machine learning is a subset of AI that focuses on the development of algorithms that allow computers to learn from and make predictions based on data. By using statistical techniques, ML algorithms identify patterns within large datasets and improve their performance over time without being explicitly programmed. Common algorithms include decision trees, support vector machines, and k-nearest neighbors.
Deep Learning: Deep learning, a specialized branch of machine learning, involves neural networks with many layers (hence “deep”). These networks, known as deep neural networks, are particularly effective for tasks such as image and speech recognition. Techniques like convolutional neural networks (CNNs) and recurrent neural networks (RNNs) have revolutionized fields such as computer vision and natural language understanding.
Neural Networks: Neural networks are inspired by the structure and function of the human brain. They consist of interconnected nodes (neurons) that process data in layers. Each neuron receives input, processes it, and passes the output to the next layer. This layered approach allows neural networks to model complex relationships in data, making them powerful tools for tasks like classification and regression.
Natural Language Processing (NLP): NLP is the branch of AI focused on the interaction between computers and human language. It encompasses tasks such as language translation, sentiment analysis, and chatbot development. Techniques in NLP include tokenization, parsing, and semantic analysis, which allow machines to understand and generate human language effectively.
These technologies do not work in isolation. For instance, deep learning models often rely on large amounts of data to train neural networks, and NLP systems may integrate machine learning to improve language understanding. Data is the lifeblood of AI, as it enables these algorithms to learn and adapt. Popular models like Google’s BERT for language understanding and OpenAI’s GPT series exemplify the integration of these technologies to create sophisticated AI applications.
Understanding these foundational technologies and techniques is crucial for grasping how AI functions and continues to evolve, driving innovation across various industries.
Ethical Considerations and Future of AI
The ethical implications of artificial intelligence (AI) remain a pivotal area of concern as technology continues to evolve. One significant ethical issue is job displacement. As AI systems become more capable, they may replace human roles in various industries, potentially leading to widespread unemployment. This shifts the labor market dynamics, requiring a balance between technological advancement and workforce retraining to mitigate adverse effects.
Privacy concerns also emerge prominently in the discourse on AI ethics. AI systems often rely on vast amounts of data to function effectively, raising questions about data security and individual privacy. The potential misuse of personal data by AI-driven applications necessitates robust data protection regulations and transparent data handling practices to safeguard user information.
Another critical ethical consideration is decision-making biases. AI models trained on biased datasets can perpetuate and even amplify existing prejudices, leading to unfair outcomes. This highlights the need for developing AI systems that are both fair and transparent, ensuring equitable treatment across all demographics. Ongoing debates emphasize the importance of incorporating ethical guidelines in the AI development process to address these biases.
To ensure responsible AI development, various measures are being taken globally. Regulatory frameworks, ethical guidelines, and interdisciplinary research initiatives aim to promote AI that aligns with societal values. These efforts include establishing AI ethics committees, implementing ethical AI principles, and fostering collaboration between tech developers, policymakers, and ethicists.
Looking ahead, the future of AI holds immense potential. Advancements in technology are likely to introduce new applications across sectors such as healthcare, education, and transportation. However, the long-term impact of AI on society will depend on how effectively ethical considerations are integrated into its development. Balancing innovation with ethical responsibility is crucial to harnessing the benefits of AI while minimizing potential risks.